Total Productive Maintenance (TPM): The Ultimate Guide to AI-Powered Performance
May 26, 2025
Total Productive Maintenance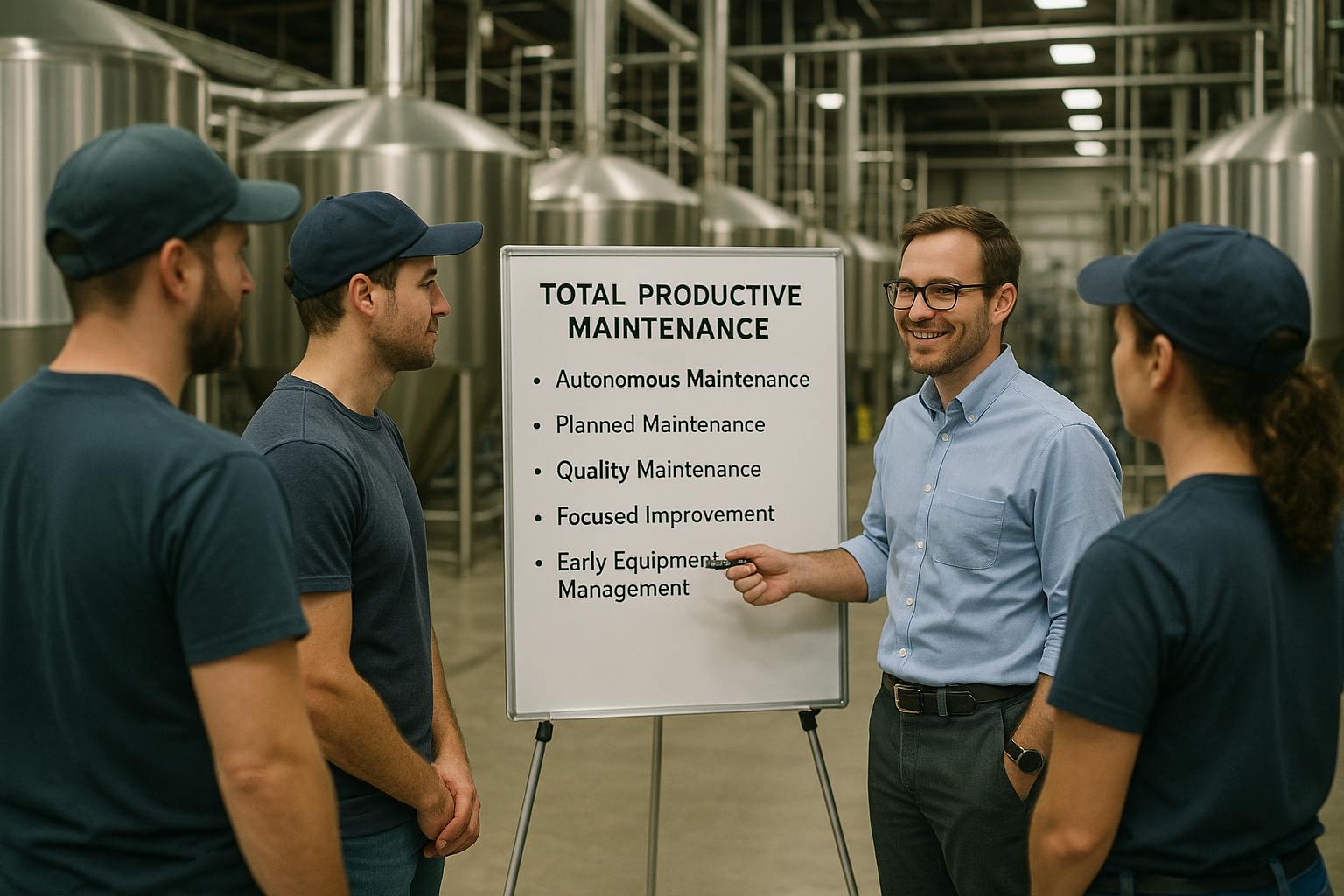
In the relentless pursuit of manufacturing excellence, where every second of uptime and every percentage point of yield matters, few philosophies have proven as powerful or enduring as Total Productive Maintenance (TPM). Born from the crucible of post-war Japanese industry and perfected by pioneers like the Nippondenso (part of Toyota), TPM is a transformative strategy that fundamentally redefines the relationship between people and machines. It’s a holistic, company-wide approach built on a simple, profound idea: everyone in the organization, from the C-suite to the shop-floor operator, shares responsibility for the health and performance of the equipment.
The goal is audacious and absolute: the complete elimination of loss. This means no breakdowns, no small stops, no defects, and no accidents. The universal benchmark for this state of manufacturing perfection is Overall Equipment Effectiveness (OEE).
For decades, companies across the globe have used the principles of TPM and its eight pillars to drive remarkable, life-altering improvements in their operations. Yet, in the age of the smart factory, many organizations find their TPM initiatives stagnating. They hit a wall, bogged down by a sea of paper checklists, cumbersome manual data entry, and time-lagged decisions. The powerful spirit of TPM is willing, but the traditional, analog methods are weak.
This is where the revolution begins.
The fusion of Artificial Intelligence (AI), the Internet of Things (IoT), and modern, AI-native Computerized Maintenance Management Systems (CMMS) is breathing supercharged new life into every facet of TPM. This isn't just an upgrade; it's a paradigm shift. It’s transforming TPM from a manual, philosophy-driven exercise into a dynamic, data-powered strategy that can finally deliver on the ultimate promise of zero losses.
This comprehensive guide will take you on a deep dive into the world of Total Productive Maintenance. We will provide a masterclass in its foundational principles, the cultural bedrock it’s built upon, and the 8 pillars that form its structure. But crucially, we will show you how to break free from the constraints of the past and leverage AI to build a TPM ecosystem that is predictive, intelligent, and relentlessly effective in the 21st century.
What is Total Productive Maintenance (TPM)?
At its core, Total Productive Maintenance (TPM) is a company-wide strategy focused on maximizing the effectiveness of equipment throughout its entire lifecycle. It achieves this by dismantling the traditional, often adversarial, walls between departments. In a TPM environment, the maintenance team are no longer the "fixers" who are only called when something is broken. Operations teams are no longer just "users" of the equipment.
Instead, TPM integrates them into a single, cohesive force with a unified goal. Operators are empowered and trained to proactively maintain their own machines. Maintenance teams are elevated from firefighting to executing strategic, data-driven preventive activities and leading complex improvement projects. Engineers are tasked with designing new equipment that incorporates the collective knowledge of the organization, making it more reliable, safer, and easier to maintain from day one.
The Bedrock of TPM: 5S and a Culture of Ownership
Before you can even begin to construct the eight pillars of TPM, you must lay a solid foundation. In TPM, that foundation is 5S. 5S is a systematic methodology for creating and maintaining an organized, clean, and efficient workplace. It’s a fundamental prerequisite because it establishes the discipline and visual control necessary for TPM to succeed.
The five steps are:
- Seiri (Sort): Go through the workspace and remove everything that is not needed for the current production process. This means clearing out old tools, unnecessary parts, and personal items. The guiding principle is: "When in doubt, throw it out."
- Seiton (Set in Order): Arrange all necessary items so they are easy to find, use, and return. This is about "a place for everything, and everything in its place." This involves creating shadow boards for tools, clearly labeling storage locations, and designing ergonomic workstations.
- Seiso (Shine): Clean the workplace thoroughly. This is not just about aesthetics. In a TPM context, cleaning is a form of inspection. As operators clean their equipment, they can easily spot leaks, loose bolts, cracks, and other early signs of trouble.
- Seiketsu (Standardize): Create standards and procedures to ensure the first three S's are maintained. This involves developing checklists, documenting best practices, and using visual aids to make the standards clear and easy to follow.
- Shitsuke (Sustain): Build the discipline to maintain the standards over the long term. This is the most challenging step and requires consistent leadership support, regular audits, and embedding the 5S mindset into the company culture.
Without a strong 5S foundation, a TPM initiative is doomed to fail. You cannot expect an operator to spot a small oil leak on a machine that is already caked in dirt and surrounded by clutter. 5S creates the baseline of stability and order from which all other improvements can grow.
The North Star of TPM: Mastering OEE (Overall Equipment Effectiveness)
You cannot improve what you cannot measure. The ultimate metric for the success of a TPM program, its unwavering north star, is Overall Equipment Effectiveness (OEE). OEE is the gold standard for measuring manufacturing productivity, distilling all the potential equipment-related losses that rob you of your capacity into a single, powerful score.
OEE is calculated as the product of three critical factors, each representing a different category of loss:
OEE = Availability x Performance x Quality
Let’s break this down:
- Availability: This measures time-based losses. It compares the time the machine was scheduled to run with the time it actually ran. Availability is reduced by Downtime Losses.
- Performance: This measures speed-based losses. It compares the number of units the machine could have produced in its run time with the number it actually produced. Performance is reduced by Speed Losses.
- Quality: This measures defect-based losses. It compares the total number of units produced with the number of good, sellable units. Quality is reduced by Quality Losses.
A world-class OEE score is typically cited as 85% or higher, but the real power of OEE is not just the final number; it's the insight it provides through its components.
The Six Big Losses: The Enemies of OEE
TPM aims to systematically eliminate the "Six Big Losses," which are the underlying causes of poor OEE.
Losses that affect Availability:
- Equipment Breakdowns: Any unplanned stop due to machine failure. This is the most visible and disruptive form of loss.
- Setup and Adjustments: Planned downtime for product changeovers, tool changes, or major adjustments. While planned, TPM seeks to drastically reduce this time through techniques like Single-Minute Exchange of Die (SMED).
Losses that affect Performance: 3. Idling and Minor Stoppages: Short stops where the machine halts for a brief period but doesn't require maintenance intervention (e.g., a jammed sensor, a misaligned part). These are often "invisible" losses that operators learn to live with. 4. Reduced Speed: When equipment runs slower than its ideal, designed cycle time. This can be due to poor lubrication, worn tools, or fear of producing bad parts at higher speeds.
Losses that affect Quality: 5. Process Defects: Producing scrap or parts that need to be reworked. These defects are caused by inconsistent process parameters or equipment malfunctions. 6. Reduced Yield During Startup: Defects produced in the initial phase of production after a startup or changeover, before the process has stabilized.
By categorizing every minute of lost production into one of these Six Big Losses, a TPM program can identify its biggest enemies and attack them with surgical precision.
The 8 Pillars of TPM: A Modern Framework for Excellence
TPM is built on a foundation of eight interconnected pillars. Each pillar represents a specific set of activities and a focus area. We will explore each pillar through two lenses: its core, traditional principle and its revolutionary potential when supercharged by AI and a modern CMMS like Factory AI.
1. Autonomous Maintenance (Jishu Hozen)
Traditional Approach: This is arguably the most revolutionary pillar of TPM. It transfers routine maintenance responsibilities from the maintenance department to the machine operators. The goal is to empower operators to become the first line of defense for their equipment. This is a multi-step process that evolves over time:
- Step 1: Initial Cleaning and Inspection.
- Step 2: Eliminating Sources of Contamination and Improving Accessibility.
- Step 3: Developing Standards for Cleaning, Inspection, and Lubrication (CIL).
- Step 4: General Inspection Training.
- Step 5: Autonomous Inspection and Monitoring.
- Step 6: Standardization of All Workplace Procedures.
- Step 7: Full Autonomous Management.
This process, managed with paper checklists and visual aids, aims to prevent forced deterioration and allows operators to detect abnormalities early.
The Factory AI Revolution: AI transforms Autonomous Maintenance from a set of manual chores into an intelligent, data-driven function.
- Digital CIL and Guided Workflows: The static paper checklist is replaced by a dynamic workflow on a tablet or mobile device, powered by the Factory AI CMMS. The system presents the operator with daily tasks, complete with visual aids, instructional videos, and required data entry fields (e.g., "Enter pressure gauge reading").
- AI-Assisted Anomaly Detection: An operator notices a slight change in the machine's sound. They use the app to record a short audio clip. The AI compares this clip to a baseline "healthy" signature and can immediately flag it as a potential anomaly, perhaps cross-referencing it with a slight increase in motor temperature that a sensor has also detected. The AI might suggest, "Vibration signature indicates potential bearing wear in Drive Motor 3. Create a high-priority inspection request for maintenance?"
- Empowerment Through Data: The operator's dashboard doesn't just show production numbers. It shows real-time equipment health data—temperature, vibration, energy consumption—in an easy-to-understand format. An alert might pop up saying, "Hydraulic fluid temperature is 7% above normal for the current load. Check for blocked ventilation or low fluid levels." This turns the operator into a highly informed, proactive guardian of the asset, not just a cleaner.
2. Planned Maintenance
Traditional Approach: This pillar establishes a systematic approach to maintenance activities, aiming to eliminate unplanned breakdowns entirely. It involves:
- Analyzing historical breakdown data to understand failure patterns.
- Creating time-based (TBM) and usage-based (UBM) preventive maintenance (PM) schedules.
- Managing spare parts inventory.
- Budgeting for maintenance activities. The goal is to increase Mean Time Between Failures (MTBF) and reduce Mean Time To Repair (MTTR), managed within a traditional CMMS or spreadsheets.
The Factory AI Revolution: This is where AI delivers its most dramatic and financially significant impact. Planned Maintenance evolves into Predictive Maintenance (PdM) and AI-Prescriptive Maintenance.
- From Calendar to Condition: The AI-native CMMS ingests data from IoT sensors monitoring vibration, thermography, ultrasonics, and other condition indicators. A PM task is no longer scheduled because "it's the first of the month." It's triggered because the AI algorithm detects the actual, real-world condition of the asset is degrading.
- Predictive Work Orders: The Factory AI platform detects the faint, early-stage signature of a gear tooth crack inside a critical gearbox. It analyzes the rate of degradation and predicts that the gearbox has a 95% probability of failing in the next 450 operating hours. This is not a guess; it's a data-driven forecast. The system automatically generates a predictive work order, checks inventory for the required rebuild kit, and allows the planner to schedule the repair for the next planned facility-wide downtime, completely avoiding a catastrophic, production-halting failure.
- AI-Driven PM Optimization: Traditional PM programs are notoriously inefficient, often including tasks that add no value. AI can analyze years of PM history and failure data. It might discover that a specific monthly lubrication task on a set of bearings has never once been correlated with preventing a failure. The system can then recommend eliminating this task, freeing up hundreds of technician hours per year for more value-added work.
3. Quality Maintenance (Hinshitsu Hozen)
Traditional Approach: This pillar focuses on ensuring the equipment is capable of consistently producing zero-defect products. It involves identifying and eliminating the root causes of quality defects by analyzing the relationship between equipment conditions and quality outcomes. The goal is to move from "detecting" bad parts to "preventing" them from ever being made. This is typically done through manual statistical process control (SPC) and offline analysis.
The Factory AI Revolution: AI enables real-time, predictive quality control by directly linking process conditions to quality outcomes.
- AI-Powered Root Cause Correlation: The Factory AI platform ingests data from two critical streams: machine process data (e.g., temperatures, pressures, speeds, torque) and quality output data from vision systems, scanners, or CMMs. The AI's machine learning algorithms can then identify the complex, multi-variable "recipe" for a defect. It might discover that 90% of cosmetic surface blemishes occur when the injection molding machine's nozzle temperature is above 215°C and the hydraulic clamp pressure fluctuates by more than 2% and the raw material moisture content is above a certain threshold. A human could never find this correlation in a spreadsheet.
- Predictive Quality Alerts: With this knowledge, the system can monitor the live process parameters and alert operators before a defect is about to be produced. The alert on the HMI might read, "Warning: Process parameters are drifting towards a known defect condition. Reduce nozzle temperature by 3°C to maintain quality." This is the holy grail: preventing the creation of scrap and rework in the first place.
4. Focused Improvement (Kobetsu Kaizen)
Traditional Approach: This pillar is about continuous, incremental improvement. It involves forming small, cross-functional teams (operators, maintenance, engineers) to tackle specific, recurring problems, typically the "Six Big Losses" identified by OEE analysis. These Kaizen teams use structured problem-solving tools like Root Cause Analysis (RCA), fishbone diagrams, and the "5 Whys" to develop, test, and implement improvements.
The Factory AI Revolution: AI acts as a super-powered data analyst for the Kaizen team.
- Automated Opportunity Finding: The Factory AI platform acts as a tireless watchdog, continuously analyzing OEE, downtime, and micro-stoppage data. Instead of waiting for a monthly steering committee meeting, the system can automatically generate a daily or weekly "Top 5 Losses" report. It can alert the Kaizen team leader: "Minor stops on Packaging Line #4 accounted for 47 minutes of lost production yesterday, making it the single biggest performance loss in the facility. The most frequent cause was 'Photo Eye Sensor Blocked'." This provides the team with a data-rich, pre-analyzed, and prioritized starting point for their efforts.
- Accelerated Root Cause Analysis: A Kaizen team investigating a recurring fault can query the AI. "Show me all process parameters for the 5 minutes leading up to the last 10 instances of this failure." The AI can instantly overlay graphs of temperature, pressure, vibration, and speed, revealing a hidden pattern that would have taken days of manual data-logging to find.
5. Early Equipment Management
Traditional Approach: This pillar aims to make new equipment "vertically start-up," meaning it performs perfectly from day one. It achieves this by applying the collective knowledge gained from maintaining existing equipment to the design and installation of new machines. The goal is to design equipment that is easier to operate, clean, inspect, and maintain. This traditionally relies on compiling "lessons learned" documents and design review checklists.
The Factory AI Revolution: The AI-native CMMS becomes a dynamic, searchable library of real-world equipment knowledge.
- Data-Driven Design for Reliability: When designing a new production line, engineers can query the Factory AI platform: "For all assets in the 'CNC Machining' class, what were the top 10 components that caused the most downtime over the last 5 years?" The system can instantly provide a detailed analysis of failure modes, MTBF for specific components from different manufacturers, and associated maintenance costs. This allows engineers to design out the known problems of the past by specifying more robust components or designing for easier access and maintenance.
- Flawless Startup and Commissioning: Based on the new design, the system can automatically generate a comprehensive "digital twin" of the maintenance requirements. It can create the asset hierarchy in the CMMS, recommend the initial spare parts list based on predictive failure models, and generate the initial PM schedule and operator CIL tasks before the machine is even uncrated. This ensures a smooth, rapid, and data-driven commissioning process.
6. Training and Education
Traditional Approach: This pillar focuses on systematically upgrading the skills of all employees to support the TPM goals. It involves creating a skills matrix, identifying gaps, and providing training for operators in autonomous maintenance tasks, for maintenance technicians in advanced diagnostics, and for managers in TPM leadership.
The Factory AI Revolution: AI provides personalized, just-in-time training and knowledge transfer.
- Augmented Reality (AR) Guided Work: A less experienced technician is assigned a complex repair. They can use a tablet or AR headset to overlay digital work instructions directly onto the machine. The Factory AI system can guide them step-by-step, showing which bolts to loosen, highlighting the specific component to replace, and providing torque specifications, all in their field of view.
- Dynamic Skills Gap Analysis: The system can track the first-time fix rate and time-to-complete for different technicians on different types of jobs. This data can help a maintenance manager identify specific skills gaps within the team without bias. The manager can then assign targeted online training modules or pair team members for on-the-job training, ensuring the entire workforce is continuously improving.
7. Safety, Health, and Environment (SHE)
Traditional Approach: This pillar aims to create a zero-accident workplace by integrating safety into the core of all TPM activities. It involves identifying and eliminating potential safety risks through job hazard analysis, safety audits, and establishing safe working procedures. The goal is an incident-free workplace.
The Factory AI Revolution: AI becomes a proactive safety guardian, predicting and preventing unsafe conditions.
- Predicting Unsafe Conditions: An AI model can learn the signature of an impending catastrophic failure. For example, it could detect an unusual energy spike combined with a specific acoustic signature from a large press, which indicates a mechanical binding that could lead to a major failure. The system can automatically initiate a safe shutdown of the equipment and alert the team before an accident can occur.
- Automated Compliance and Auditing: The CMMS ensures that all safety-critical PMs, fire extinguisher inspections, and safety harness checks are completed on time. It provides a permanent, fully auditable digital trail, making regulatory compliance effortless and robust.
8. TPM in Administration
Traditional Approach: This pillar applies the principles of TPM and waste elimination to administrative and support functions. It focuses on improving the efficiency of processes like procurement, production scheduling, and new product introductions.
The Factory AI Revolution: AI automates and optimizes these administrative workflows with predictive insights.
- Intelligent MRO Inventory Management: The predictive capabilities of the Factory AI platform mean it knows which critical spare parts will be needed for maintenance weeks or even months in advance. It can analyze consumption patterns and future predictive work orders to generate automated, optimized purchase requisitions. This ensures critical parts arrive just-in-time, slashing inventory carrying costs while simultaneously eliminating production delays caused by out-of-stock components.
- Optimized Production Scheduling: By providing the production scheduling system with accurate, data-driven predictions of potential equipment downtime, the AI allows for more realistic and achievable production schedules, reducing the chaos and stress caused by constant firefighting and rescheduling.
A Prospect's Journey: A Brewery's Path to TPM Excellence
To see how these principles translate from theory into a practical, forward-thinking plan, let's look at the journey of a rapidly growing brewery we're currently in discussions with. They are facing challenges familiar to many scaling manufacturers: unexpected downtime on their bottling line during peak demand, inconsistent product quality due to fluctuating fermentation temperatures, and a maintenance team that was perpetually in a state of reactive firefighting.
They knew they needed a more structured approach and have mapped out a five-stage TPM journey. Our conversations have focused on how a modern, AI-native platform can serve as the digital backbone for their ambitious vision, starting from day one.
Stage 1: Laying the Foundation (Months 1-6)
The brewery's plan for the first six months is all about establishing basic stability. They are initiating a full 5S campaign to declutter and organize their entire facility, from the brewhouse to the packaging hall. A critical part of this stage is implementing a modern CMMS to replace their chaotic system of spreadsheets and whiteboards. The goal is simple: digitize all assets and begin capturing clean data on every work order. This will allow their maintenance team to move from purely reactive work to a basic Planned Maintenance system based on manufacturer recommendations.
Stage 2: Empowering the Operators (Months 7-18)
Once a clean, organized workplace and a digital maintenance system are in place, the brewery plans to launch its Autonomous Maintenance pillar. Operators on the bottling line will be trained to perform daily cleaning, inspection, and lubrication (CIL). The vision is for them to use tablets to log their findings directly into the CMMS, creating a rich stream of data on machine health. They anticipate that this will empower operators to catch small issues—loose fittings, worn guides, minor leaks—long before they can cause a major stoppage, leading to the first significant jump in their OEE.
Stage 3: Proactive Control & PdM Consideration (Current Planning Stage)
Having achieved a new level of stability, the brewery's focus in their strategic plan shifts to tackling more complex, intermittent problems. This is where they will launch their Quality Maintenance pillar, using the data collected in the CMMS to correlate minor process deviations with quality control flags.
It's at this stage that their leadership team is strategically planning for Predictive Maintenance (PdM). They recognize the inherent limitations of time-based maintenance. They recounted a recent, costly, and unplanned rebuild on a critical gearbox in their malt mill, which occurred despite the asset having been serviced on schedule just two months prior. This event crystallized their understanding that a calendar date doesn't reflect an asset's true condition.
Stage 4: The Predictive Leap (The Envisioned Future)
The brewery's forward-looking plan for this stage is to launch a PdM pilot program as a core part of their Focused Improvement pillar. In our consultations, we've analyzed their asset list and past failures. The bottling line's main drive motor and the fermentation tank chillers—assets where failures put entire batches of their flagship IPA at risk—have been identified as prime candidates. The plan involves installing wireless vibration and temperature sensors on these assets, feeding live data into an AI platform. The goal is for the AI to learn their unique operational signatures and begin to predict failures related to bearing wear or compressor inefficiency before they can impact production. Success here, they believe, will shift their entire maintenance paradigm from prevention to prediction.
Stage 5: Optimization and Innovation (The Ultimate Goal)
Looking ahead, the brewery envisions that a successful rollout of PdM in Stage 4 will unlock the final stage of their journey. With predictive insights flowing from their critical assets, their Early Equipment Management pillar will be supercharged. When they purchase their next canning line, they will use years of data to specify components with proven reliability, essentially designing out future failures. Their goal is to have TPM fully embedded in their culture, with their CMMS evolving from a simple system of record into an intelligent engine that drives continuous, data-powered improvement across their entire operation.
The Future is Now: From Theory to AI-Powered Results
The philosophy of Total Productive Maintenance, with its focus on empowerment, ownership, and the relentless elimination of waste, is more relevant today than ever before. It provides the cultural operating system for manufacturing excellence. But to unlock its true, transformative potential in the 21st century, you must move beyond the manual, reactive methods of the past.
The future of TPM is not about more paper checklists; it's about better, real-time data. It's not about more team meetings; it's about more intelligent, data-driven insights. It's not about reacting to yesterday's failures; it's about predicting and preventing tomorrow's.
By integrating the proven principles of TPM with the analytical power of an AI-native CMMS and predictive maintenance platform, you create a dynamic, self-improving ecosystem. You empower your people with the data they need to make smarter, faster decisions. You optimize the performance and reliability of your assets beyond what was previously thought possible. And you build a sustainable, winning culture of excellence that drives directly and powerfully to your bottom line.
Don't let your TPM initiative stall. Discover how Factory AI can provide the intelligent engine to power your journey to world-class OEE.
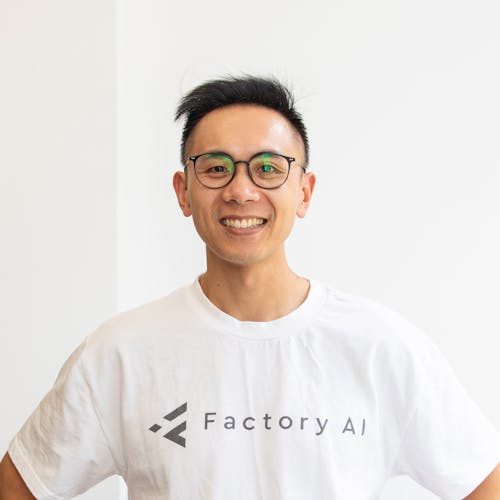
Tim Cheung
Tim Cheung is the Co-Founder of Factory AI, a startup dedicated to helping manufacturers leverage the power of predictive maintenance. With a passion for customer success and a deep understanding of the industrial sector, Tim is focused on delivering transparent and high-integrity solutions that drive real business outcomes. He is a strong advocate for continuous improvement and believes in the power of data-driven decision-making to optimize operations and prevent costly downtime.