Understanding the Differences Between Predictive Maintenance and Condition Monitoring
Jan 29, 2025
Predictive Maintenance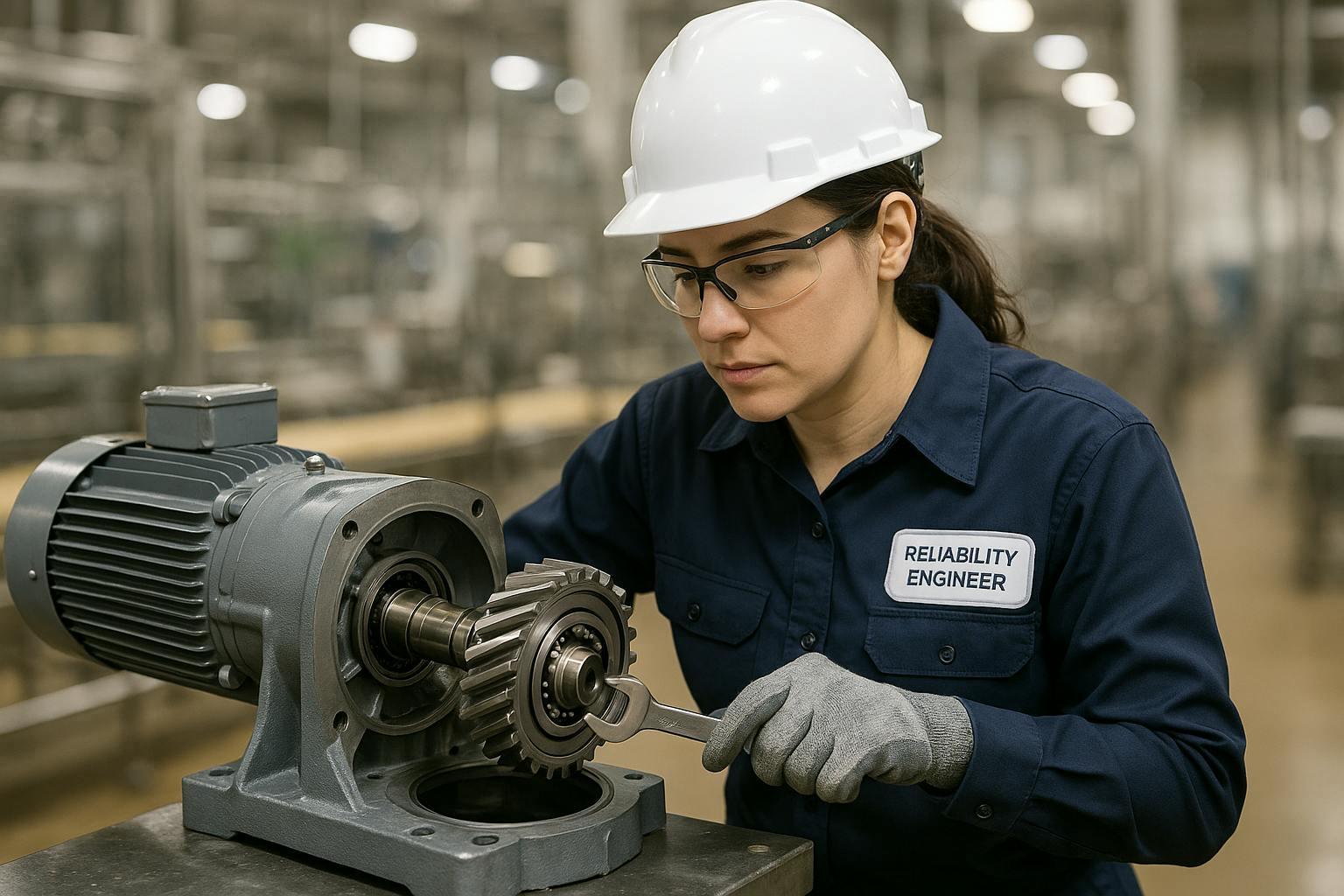
Introduction:
In the quest for optimal machine health and operational excellence, manufacturers often encounter two key strategies: predictive maintenance and condition monitoring. While these terms are sometimes used interchangeably, they represent distinct approaches to managing asset reliability. Understanding the nuances between them is crucial for making informed decisions that can significantly reduce downtime, cut costs, and enhance overall productivity. At Factory AI, we've worked with hundreds of manufacturing sites across Australia and New Zealand, gaining firsthand insights into how these strategies are applied in the real world. This article will demystify predictive maintenance and condition monitoring, explore their core differences, and help you determine which approach—or combination of approaches—is the best fit for your facility.
What is Condition Monitoring?
Condition monitoring is akin to having a real-time check-up on your equipment’s health. This strategy continuously tracks the status of machinery using sensors that measure parameters such as vibration, temperature, pressure, and oil quality. When any parameter exceeds a predefined threshold, an alert is triggered, prompting immediate maintenance action to prevent equipment failure. In most applications, condition monitoring uses threshold-based alerts rather than AI or machine learning models to identify issues.
Think of condition monitoring as a ‘here and now’ approach: it identifies issues as soon as they arise, enabling maintenance teams to respond promptly. For instance, if vibration levels in a motor suddenly spike, an alert is sent to indicate that action is required.
Pros and Cons of Condition Monitoring
Benefits:
- Provides real-time feedback on asset health.
- Minimises unexpected breakdowns.
- Helps prioritise maintenance tasks, avoiding unnecessary work.
Challenges:
- Relies on fixed thresholds, which can lead to false positives.
- Often requires human interpretation of results.
- Sensor accuracy and consistency can limit its effectiveness.
Vibration condition monitoring is one of the most common techniques within this approach, making it especially useful for rotating machinery like pumps, motors, and compressors.
Credit to Steve Hanley and the Endaq blog for this image. While our perspective differs slightly, certain principles resonate—particularly the broader data scope often utilised in predictive maintenance
What is Predictive Maintenance?
Predictive maintenance goes beyond real-time tracking by using advanced software and analytics to predict future equipment failures. By analysing patterns and trends in aggregated sensor data, predictive maintenance can forecast potential failures weeks or even months in advance.
For example, while condition monitoring may trigger an alert due to a sudden rise in temperature, predictive maintenance software can identify the trend over time, suggesting that a failure might occur in 60 days if no action is taken. The main distinction lies in how alerts are handled: condition monitoring signals the current status, while predictive maintenance provides context by identifying potential root causes, failure probabilities, and recommended actions. Some solutions, such as ours at Factory AI, leverage AI to analyse vast datasets from thousands of assets and suggest appropriate responses.
Pros and Cons of Predictive Maintenance
Benefits:
- Extends equipment lifespan by addressing issues before they escalate.
- Reduces downtime and maintenance costs.
- Delivers data-driven insights that enhance asset management.
- Provides valuable context around alerts, helping identify root causes and estimate failure probabilities.
Challenges:
- Requires significant initial investment in technology and training.
- Needs time to gather sufficient data to build accurate models.
- Implementation can be complex without the right expertise (though solutions like Factory AI simplify this process).
Predictive maintenance relies on a combination of sensors and advanced analytics, making it a more sophisticated evolution of condition monitoring. It uses machine learning to improve accuracy and reliability over time.
Another insightful (and humorous!) image from the same Endaq blog post. It’s a reminder to discuss with your vendor how they distinguish between these approaches to find the best fit for your needs
Summary of Key Differences Between Predictive Maintenance and Condition Monitoring
Here’s a closer look at the main differences:
- Timing:Condition Monitoring: Focuses on real-time changes in equipment status.Predictive Maintenance: Predicts future failures based on historical data and trends, allowing for scheduled maintenance before issues arise.
- Data Usage:Condition Monitoring: Relies on individual sensor data at a specific moment.Predictive Maintenance: Aggregates data from multiple sources, using algorithms to develop a comprehensive view of asset health.
- Action Triggers:Condition Monitoring: Initiates maintenance based on pre-set thresholds (e.g., vibration exceeding safe limits).Predictive Maintenance: Activates maintenance based on statistical predictions of potential failures.
- Implementation Complexity:Condition Monitoring: Generally simpler, focusing on straightforward data collection and alerts.Predictive Maintenance: Involves complex algorithms and often requires specialised training, though tools like Factory AI streamline the process.
- Cost and ROI:Condition Monitoring Software: Moderate costs related to sensor installation and maintenance, with quicker returns due to reduced downtime.Predictive Maintenance Software: Higher initial costs due to analytics tools but offers substantial long-term savings by enhancing equipment reliability. (In solutions like Factory AI, the upfront cost is comparable to that of condition monitoring.)
- Context:Condition Monitoring: Requires manual review of data from the CMMS and historical work orders to establish context, which can be time-consuming.Predictive Maintenance: Uses data and AI models to deliver context, solving the ‘too much data, too little insight’ problem.
How to Choose Between Condition Monitoring and Predictive Maintenance
The choice depends on various factors such as equipment type, asset criticality, and budget. Maintenance and reliability leaders often use this simple framework:
- Immediate, real-time response: Condition monitoring is ideal, especially for critical equipment requiring constant operation, like in manufacturing or utilities.
- Long-term, strategic planning: Predictive maintenance is more suitable for organisations looking to maximise uptime and performance through advanced analytics.
A hybrid approach can be particularly beneficial—using condition monitoring to detect immediate issues, while predictive maintenance supports longer-term planning. This strategy is part of why we created Factory AI, which combines simplicity and affordability with the benefits of advanced machine learning and AI.
Final Thoughts: Which Strategy Fits Your Site?
Erik Hupjé recently noted, “Many organisations invest in these new kinds of technologies to ‘improve’ reliability while neglecting basic principles... In most cases, predictive maintenance is little more than condition-based maintenance marketed with a new buzzword.”
Erik's simple visual reminds us that Predictive Maintenance is just one of nine strategies, each with its own role within your broader reliability vision.
The key takeaway is to always start with basic principles before adopting new technologies:
- Start with an assessment of where you are today; consider whether your current condition monitoring is delivering enough value to justify investing in a more advanced, complex solution.
- Challenge vendors and consultants to clarify how their solutions distinguish predictive maintenance from condition monitoring.
With the right partner, you’ll find a great solution that can unlock added value from your existing data and take it even further.
Both approaches are essential for maintaining assets, boosting efficiency, and reducing downtime. Implementing either condition monitoring or predictive maintenance requires the right tools, training, and commitment. While vibration condition monitoring can prevent immediate issues, predictive maintenance extends reliability by forecasting failures. Ultimately, choosing the right strategy can transform how you manage machine health, offering significant returns on investment.
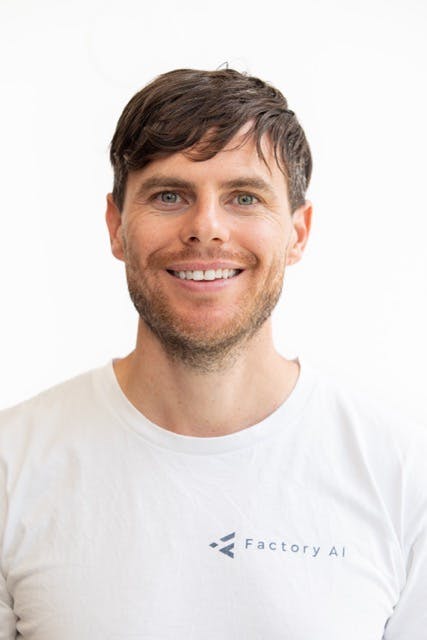
JP Picard
Jean-Philippe Picard is the CEO and Co-Founder of Factory AI. As a positive, transparent, and confident business development leader, he is passionate about helping industrial sites achieve tangible results by focusing on clean, accurate data and prioritizing quick wins. Jean-Philippe has a keen interest in how maintenance strategies evolve and believes in the importance of aligning current practices with a site’s future needs, especially with the increasing accessibility of predictive maintenance and AI. He understands the challenges of implementing new technologies, including addressing potential skills and culture gaps within organizations.