How to Get Started With Predictive Maintenance: A 12-Step Roadmap for Reliability Leaders
Apr 3, 2025
Predictive Maintenance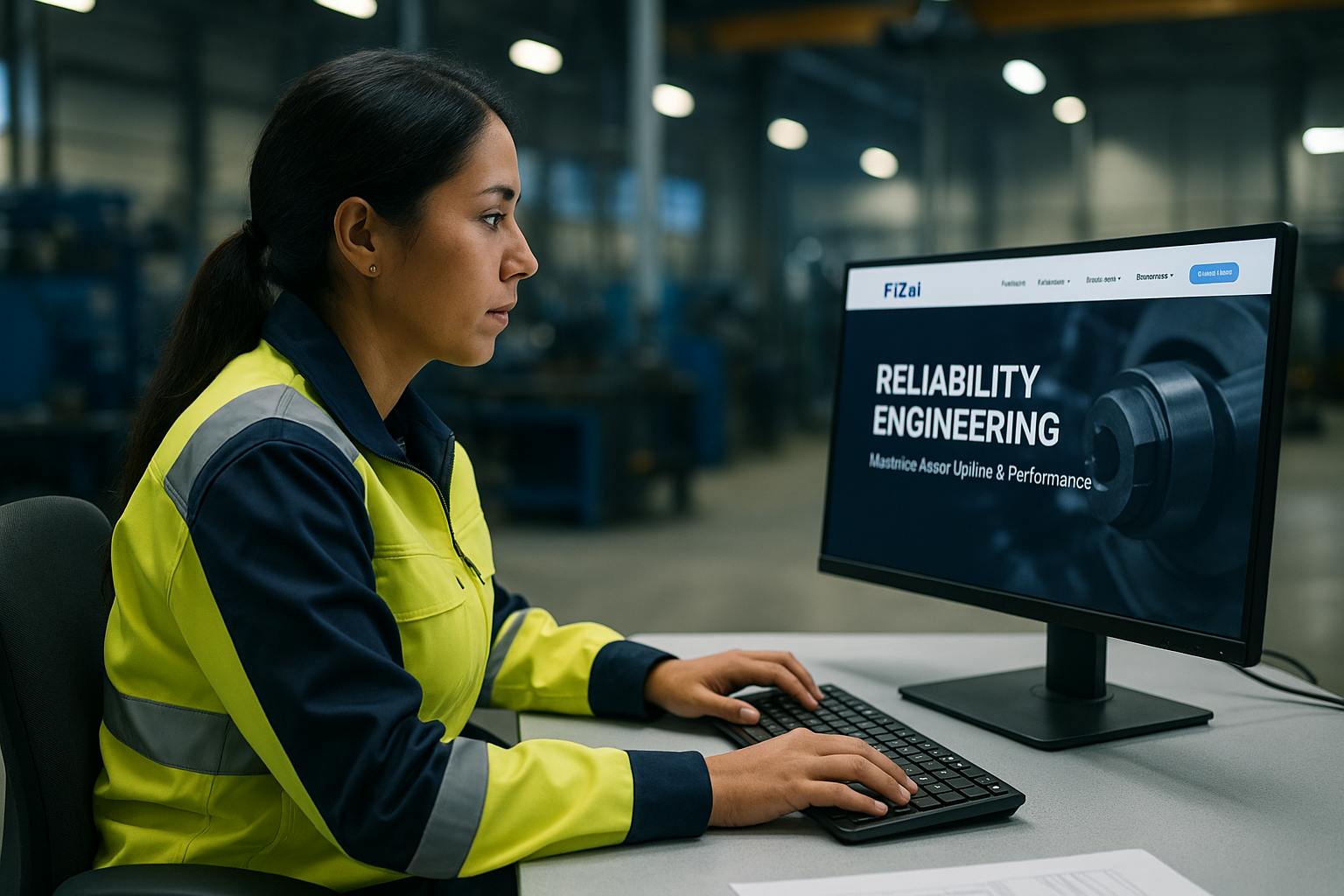
Predictive maintenance (PdM) isn't just a buzzword for large corporations anymore; it's a vital strategy for any manufacturing site aiming to slash unplanned downtime, cut costs, and boost reliability. Yet, many reliability leaders find themselves stuck, either unsure how to begin or frustrated by pilot programs that fail to scale. Does this sound familiar?
The good news is that achieving real results with PdM doesn't require a massive budget or overwhelming complexity. This 12-step guide, built on industry research and real-world experience, offers a clear path to bridge the gap between theory and practical execution. Whether you're just starting your PdM journey or looking to expand a successful pilot, these steps will help you take meaningful action and achieve tangible improvements.
What Qantas, Netflix and Norwegian Triathletes Can Teach Us About Reliability
We kicked off our recent presentation to reliability professionals with a short quiz. The answers weren’t just trivia — they tell a story about how data, experimentation, and predictive thinking create massive value in very different domains.
- Qantas saved tonnes of jet fuel not by reducing meals or luggage, but by using AI to optimise flight paths in real time.
- Billy Beane, of Moneyball fame, outperformed baseball giants by trusting stats over scouts.
- Netflix crushed Blockbuster by making data-driven decisions and delivering personalised, on-demand content.
- Norwegian triathletes like Gustav Iden and Kristian Blummenfelt dominate their sport through relentless experimentation and a scientific training approach.
What do all of these have in common? They relied on:
- Real-time data,
- Algorithms that outperformed gut feel,
- Willingness to try, test, and iterate,
- A deep focus on reliability and performance.
That’s exactly what predictive maintenance offers to manufacturers — a chance to use data, AI, and continuous improvement to run more reliably.
As W. Edwards Deming said:
"Without data, you're just another person with an opinion."
Why the Current Approach Falls Short
Despite the prevalence of time-based preventive maintenance schedules, most equipment doesn’t fail due to age. In fact:
- Only 8–23% of failures show an increasing failure rate over time.
- A whopping 77–92% of failures occur randomly, with no clear pattern.
This was proven by studies from:
- United Airlines (1968),
- Bromberg in Sweden (1973), and
- The U.S. Navy (SUBMEPP, 1982).
This aligns with the six failure patterns outlined in research by Nowlan and Heap. Their studies revealed that most equipment does not follow the predictable "bathtub curve" many engineers were taught, but instead fail in seemingly random ways.
In other words: most failures won’t be prevented by your monthly PM schedule. Instead, they require a condition-based or predictive strategy.
The Cost of Getting This Wrong
According to Senseye’s “True Cost of Downtime” report:
- Global Fortune 500 manufacturers lose over $1.5 trillion annually to unplanned downtime.
- That’s a 50% increase in just a few years.
- Downtime eats up 11% of annual revenues in many plants.
At the same time, Deloitte found that predictive maintenance can:
- Reduce breakdowns by 70%,
- Cut maintenance costs by 25%, and
- Improve productivity by 25%.
The ROI is clear. The question is: how do you start?
The 12-Step Roadmap
Here’s our full roadmap, refined through dozens of real-world pilots across food, beverage, and industrial sites:
Step 1: Look at Your Data
Before installing sensors or buying software, start with what you already have. In most cases, your current systems hold more insight than you realise.
Start by examining:
- Asset Criticality Matrix
- Downtime hours per asset
- Failure modes per asset
- Ease and cost of repair
Also consider supporting data such as:
- MTBF (Mean Time Between Failures)
- MTTR (Mean Time to Repair)
- Maintenance backlog
- Existing condition monitoring systems (even if underused)
Your goal isn’t perfect data — it’s clarity. Identify which assets hurt most when they fail and where data gaps might be limiting insight.
🔍 Tip: Look for assets with frequent emergency work orders, repetitive failures, or high downtime costs.
Step 2: Create Your Hypothesis
Predictive maintenance should start with a hypothesis. Framing your initiative this way sets expectations and helps you gain buy-in.
Use a simple 2-page format:
Page 1: Executive Summary
- Problem: High downtime, over-maintenance, reactive firefighting.
- Solution: Run a PdM pilot to detect issues earlier.
- ROI: Estimate 2–6X return based on downtime avoided and fewer unplanned interventions.
Page 2: Business Case Detail
- Downtime cost (e.g., $2,000/hour)
- Proposed investment (hardware + software)
- Expected benefits ($30K–$80K/year range)
- Plan to pilot, measure, and scale if successful
📊 Back it up with conservative assumptions — executives hate fluff, but they love clear ROI logic.
Step 3: Get a Mentor
Connect with someone who’s already implemented PdM. Whether it’s another plant in your company, a peer in the industry, or a vendor partner, their guidance will help you:
- Avoid common pitfalls
- Learn how long it really takes
- Hear real-world alert stories (good and bad)
- Understand how they won trust with their team
Ask questions like:
- What made you decide to implement PdM?
- What challenges did you face?
- How accurate are the predictions?
- What would you do differently?
Pro tip: Ask them to share what they wouldn’t do again. Those lessons are gold.
Step 4: Select the Right Assets
Choosing the right assets can make or break your pilot.
Use these criteria:
- High impact: Downtime hurts production or costs big $$$
- Measurable degradation: Assets show signs before failing (via vibration, temperature, etc.)
- Repeatable profile: Equipment operates at consistent speed or load
- Sensor-friendly: Easy to install sensors during planned shutdown
Focus on rotating equipment like motors, pumps, fans, compressors, gearboxes. These make up a significant portion of plant failures — and they're typically well-suited to PdM.
Don’t just pick the most critical assets — include some that fail frequently. They’ll give you faster validation and build confidence.
Step 5: Get a Small Amount of Money
You don’t need a huge CapEx budget to start. One of the most common questions we hear is: “How much will it cost to try this?” Naturally, costs will vary depending on the hardware option you choose and the size of the pilot, but to offer transparency, we’ve shared below the actual cost one site paid for a 3-month pilot. While this won’t apply to every situation, it should give you a useful ballpark figure to start the conversation.
Here’s an example starter pack:
- 25 vibration/temp sensors @ $230 = $5,750
- 2 gateways @ $280 = $560
- Software: $1,000/month x 3 = $3,000
- Install and training: Often included in pilot
Total = $9,320 (GST incl.)
This gets you real-time data, automated insights, and a way to test your hypothesis.
💡 You’re solving a $100K+ problem with a $9K experiment. That’s a no-brainer ROI.
Step 6: Assemble Your SWAT Team
PdM is a team sport.
You’ll need a small, cross-functional crew to run and support the pilot:
- Site leader or sponsor – Removes roadblocks, provides air cover
- Reliability engineer – Oversees asset selection, FMEA input, alert triage
- Data analyst or tech lead – Handles sensor data, integrates with CMMS, calculates ROI
Schedule quick weekly huddles. Keep the energy up. Celebrate progress early and often.
Step 7: Integrate Alerts Into Workflows
Don’t let alerts sit in dashboards. Make sure they trigger real action:
- Connect alerts to your CMMS or work order system
- Define SOPs for triaging and responding to alerts
- Assign owners for each alert type
- Run a mock alert to test the process
Technology doesn’t change outcomes — workflow does.
Step 8: Validate and Tune the System
Track every alert:
- Was it a true positive?
- How early did it come?
- What action was taken?
Use this data to:
- Build confidence with technicians and engineers
- Fine-tune alert thresholds
- Improve future predictions
Also log missed detections or false positives. These teach you just as much.
Step 9: Scale to Additional Assets
Once you’ve had your first win, scale up smartly:
- Prioritise similar equipment types to reuse models
- Expand to other critical or failure-prone areas
- Avoid taking on too many assets at once
Scaling should feel like momentum, not overload.
Step 10: Train & Engage the Broader Team
Bring your site along for the ride:
- Share alert stories in shift meetings or toolbox talks
- Create a PdM "cheat sheet" for frontline staff
- Involve techs in failure mode analysis and alert reviews
Adoption grows when the team feels ownership.
Step 11: Report Impact and ROI
You need to prove it’s working:
- Downtime avoided
- Maintenance cost reductions
- Emergency work orders reduced
Use dashboards or simple graphs to make this visible. Pair numbers with real stories.
Step 12: Institutionalise and Improve
Make predictive maintenance part of "how we do maintenance":
- Add PdM metrics to your site KPIs
- Run quarterly reviews with leadership
- Continuously reassess: Are we targeting the right assets? Are we getting value?
Final Thoughts
Most reliability engineers live in a constant juggling act: battling reactive work, planning PMs, firefighting unplanned failures. PdM offers a way to flip that script.
But don’t fall into the trap of thinking you need to “boil the ocean.” Start small. Start where it matters. Build trust. Prove it works. Then scale.
Predictive maintenance isn’t a technology problem. It’s a leadership opportunity.
If you’re ready to run your first PdM pilot — or if you’ve tried before and want to do it better — we’re here to help.
Book a time to chat with us here: https://calendar.app.google/7gU5kMmxTscgefC37
Here’s to fewer breakdowns, more predictability, and a happier maintenance team.
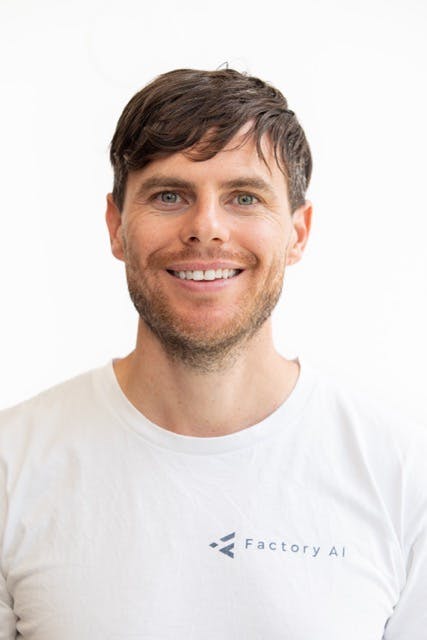
JP Picard
Jean-Philippe Picard is the CEO and Co-Founder of Factory AI. As a positive, transparent, and confident business development leader, he is passionate about helping industrial sites achieve tangible results by focusing on clean, accurate data and prioritizing quick wins. Jean-Philippe has a keen interest in how maintenance strategies evolve and believes in the importance of aligning current practices with a site’s future needs, especially with the increasing accessibility of predictive maintenance and AI. He understands the challenges of implementing new technologies, including addressing potential skills and culture gaps within organizations.